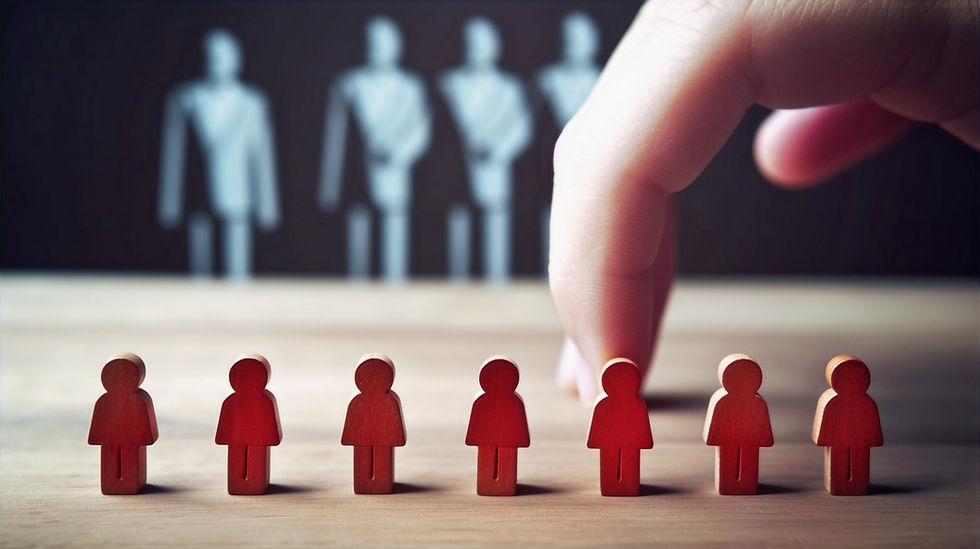
Choosing the right person for your team is a tough job, even more so when you're dealing with complex fields like AI and Computer Vision.
It's when you make a bad hire that the real problems start. Suddenly, you find yourself losing hours of precious time, watching your budget disappear, and dealing with damage to your company's reputation.
The worst part? This one mistake can stop your company's progress in its tracks. As the old saying goes, "Leaders spend 10% of their time hiring and a whopping 90% fixing hiring mistakes."
In this article, we'll talk about the missteps that can lead to bad hires in the world of AI and Computer Vision. We'll help you understand these pitfalls so you can avoid them and make your hiring process even stronger
Not Having a Clear Vision
The first hurdle in hiring for Computer Vision roles actually arises before the hiring process even starts. Computer Vision is a complex field with many nuances. If you're not clear on what you're looking for, you risk making a bad hire before you've even begun.
Key factors to consider include the technology stack you need, the exact role you're looking to fill, the candidate's past experiences, and the complexity of your computer vision problems.
For instance, knowing whether you need the fast-paced ecosystem of PyTorch or a more stable, production-ready solution like TensorFlow is crucial. Similarly, understanding the differences between a Computer Vision Engineer and a Computer Vision Scientist can help ensure you hire the right person for the job.
Also, it's important to remember that Computer Vision is a wide field with a vast range of applications. So, someone who's excelled in surveillance projects might not be the best fit for a BioTech startup, despite their impressive track record. And, your project's complexity should guide you in deciding whether you need a senior engineer or if an intern can get the job done.
In conclusion, it's vital to thoroughly assess your needs, the current status of your project, and your future plans. If you don't, you risk stumbling at every step of the hiring, onboarding, and employment process
How to Define What You Want:
If you already have a Computer Vision team, ask them to prepare a report outlining the current state and needs of your Computer Vision tasks. Also, collaborate with your business team to set hiring criteria.
If you lack the technical knowledge to decide on the factors mentioned, consider hiring a consultant. They can help identify your Computer Vision needs and guide you in the right direction.
The Pitfalls of Ambiguous Job Descriptions
An unclear and generic job description can lead to a flood of irrelevant resumes, causing you to miss out on truly qualified Computer Vision talent. Moreover, a poorly written job description might make talented individuals hesitant to apply, as it could give the impression that your company lacks a strong AI culture and maturity.
One common mistake in crafting job descriptions is asking for proficiency in all aspects of computer vision. As mentioned before, the field of Computer Vision is extensive, and it's unrealistic to expect a single person to master all its elements. The pace of evolution in the Computer Vision field is rapid, making it challenging for anyone to stay abreast of all the latest developments.
Therefore, it's crucial to be precise about the skills and tools you currently need. Look for candidates who have relevant experience and display a passion for learning new things. This way, you can be sure they'll be able to adapt swiftly as new tools or concepts are introduced in your company.
Another pitfall is designing a job description that reflects your company's aspirations more than its present needs. Your job post should focus on the skills required for the current projects, rather than those you might need in three years. Overstating or inflating requirements can lead to hiring overqualified candidates who may become bored quickly or might expect a career progression that your company can't provide.
In summary, a good job description for Computer Vision roles should be concise, specific, and realistic. It should clearly outline the required skills, tools, and experiences without being overly ambitious.
The Perils of Ineffective Screening and Assessment
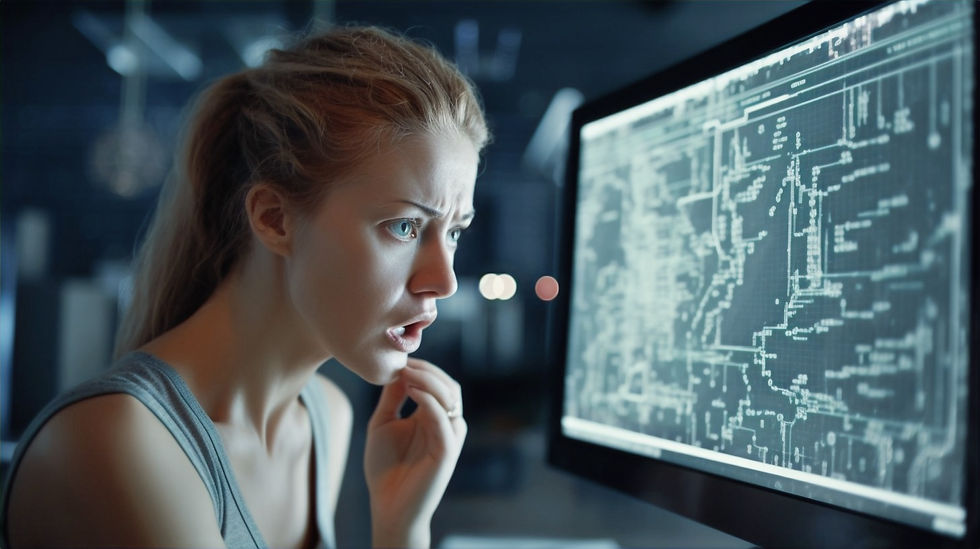
The journey to identify the perfect talent from a massive pool of resumes is undoubtedly challenging and time-intensive. An essential part of the hiring process, screening and assessment, if done poorly, can lead to disappointing results.
One common mistake during interviews is skewing too far towards either very basic or highly specific questions. Asking what KNN (K-nearest neighbors) is might be too elementary, something anyone with a basic machine learning course could answer. In contrast, inquiring about the number of layers in a YOLO (You Only Look Once) model doesn't necessarily gauge a candidate's qualifications accurately. Instead, questions should probe a candidate's understanding of how computer vision systems work, their bottlenecks, and strategies for optimization. It should also assess their ability to come up with fast, creative solutions, whether it is an open source and out-of-the-shelf solution or a custom solution.
Another challenge is assessing the coding skills of candidates. Traditional algorithm and data structure live coding tests are common approaches to gauge a candidate's problem-solving skills and comfort with a programming language.
However, in the age of AI models like ChatGPT, which can solve algorithmic problems in seconds, relying solely on these tests may not accurately measure a candidate's capabilities. Instead, providing tests that reflect daily tasks a candidate might encounter is more insightful. For instance, given a computer vision codebase, asking the candidate to improve a module such as data preprocessing or a training pipeline could provide a more accurate assessment, as it would measure both their programming and computer vision skills.
The Absence of an AI Culture
Think you've crossed the finish line once you've accurately identified your computer vision needs, crafted the perfect job description, attracted top talent, and executed a thorough assessment procedure? Not so fast! If your company lacks a solid AI culture, you could run into a variety of problems, leading to a frustrated and dissatisfied computer vision specialist.
Understanding AI and computer vision tools and systems isn't like understanding other software tools. Everyone involved in your organization should have a basic understanding of how a computer system operates in general, the inherent limitations of such systems, what you can realistically expect from a computer vision system, the time frame for a project to reach maturity, and the process of developing computer vision tools.
AI and computer engineering differ in several ways. Most computer engineering problems have a wealth of experience and best practices behind them, making it easy to estimate the development and deployment time for features like authentication or landing page creation. But AI, especially in real-world applications, is a newer field and has more uncertainties. This field relies heavily on hypotheses and experiments, making timelines for feature deployment less predictable.
Another issue with not fully understanding computer vision is the potential for unrealistic expectations. Despite significant advancements in recent years, the field still has its limitations and doesn't perform at human capacity. Unrealistic expectations can create stressful situations for your team and potentially lead to overpromising features to customers.
By clearly explaining these differences and the characteristics of AI development, stakeholders can set realistic expectations. When everyone is on the same page, it makes progress smoother for your team. Meanwhile, the company can align its goals and visions based on this mutual understanding.
Conclusion
Avoiding costly hiring mistakes in the complex field of AI and Computer Vision is no easy feat, but it's crucial to get it right. The potential pitfalls are many, from not clearly defining what you want, to crafting ineffective job descriptions, to implementing inadequate screening processes, and finally to the often overlooked aspect of not fostering an AI-centric culture.
But fret not, all these challenges can be overcome with the right knowledge, strategies, and expertise in the AI and Computer Vision field. That's exactly where TalentPulse.ai comes into play. Remember, in the field of AI and Computer Vision, a successful hire isn't just about filling a role today. It's about investing in a talent who will grow with your company, and help you navigate the exciting yet challenging landscape of AI
Computer Vision Recruitment with TalentPulse.ai
At TalentPulse.ai, we bring together a rich expertise in AI and Computer Vision, coupled with a deep understanding of the hiring landscape in these specific fields. We can help you navigate this intricate process, from defining your needs, drafting precise and effective job descriptions, implementing robust screening and assessment procedures, to cultivating an AI culture within your organization. This ensures that you hire not only the right talent but also retain them by providing a nurturing and understanding work environment.
Book a consultation for your Computer Vision Recruitment needs now!
コメント